In recent years, artificial intelligence (AI) has been used to improve the precision of valvular heart disease diagnosis and treatment. It has the ability to identify and risk stratify patients with valvular heart disease and holds promise in improving the innovation of new treatments through shorter, safer and more effective clinical trials. AI can help to guide the treatment of patients with valvular heart disease, by aiding in optimal device selection for transcatheter valvular interventions and, potentially, predicting the risk of specific complications. This review article explores the various potential applications of AI in the diagnosis and treatment of valvular heart disease in more detail.
Introduction
Medicine has benefited from increasingly advanced diagnostic and therapeutic options, which enable more tailored patient-specific strategies, with improvements in both efficacy and safety. Artificial intelligence (AI) was first researched in 1955 when John McCarthy proposed a project that attempted to “make machines use language, form abstractions and concepts, solve kinds of problems now reserved for humans, and improve themselves.”1 In the 1970s, a new probabilistic model was developed that could simulate the process of expert decision-making by assigning weight to every clinical finding to indicate its possibility of occurrence for a certain disease.2 This model was applied to machine learning that allowed computers to learn patterns from data and perform tasks without explicit programming, thus, producing an ‘artificial neural network’.3 Since then, robust risk prediction models have been developed. In healthcare, AI has, thus far, mainly revolved around machine and deep learning. Machine learning uses algorithms that enable AI to learn patterns from data and make predictions, while deep learning is a subset of machine learning that involves neural networks modelled on the human brain. Neural networks use interconnected nodes in order to process information and then represent data through a hierarchy of increasingly complex patterns.3
The development of deep learning has led to interest in the use of AI for the detection and treatment of conditions across a wide spectrum of medicine, including cardiology. In recent years, AI has been used to improve the precision of valvular heart disease diagnosis by enhancing the recognition of abnormalities in clinical examination, electrocardiography (ECG) and echocardiography. As well as playing a role in the diagnosis of patients with valvular heart disease, AI can also be used to identify those who are at highest risk of morbidity and mortality. Finally, AI can help to guide the treatment of patients with valvular heart disease, by aiding in optimal device selection for transcatheter valvular interventions and, potentially, predicting the risk of specific complications.
This review article explores the various potential applications of AI in the diagnosis and treatment of valvular heart disease in more detail.
AI-guided diagnosis of valvular heart disease
One of the major strengths of AI is its ability to decipher clinical signs that might otherwise be missed or misinterpreted by a physician. In a blinded, prospective study of 369 patients aged over 50 years without previous history of valvular heart disease, a diagnostic accuracy comparison was made between the auscultation of heart sounds by primary-care physicians using a standard stethoscope, and auscultation by a digital stethoscope with analysis by AI using deep-learning technology. The findings were compared with subsequent transthoracic echocardiography, and AI was shown to be over twice as sensitive at detecting audible valvular heart disease (corresponding to moderate or greater disease) than physicians (94.1% vs. 41.2%), with similar specificity (84.5% vs. 95.5%). The authors did not report whether AI more accurately diagnosed specific valvular lesions.4 While these results suggest AI’s superiority to the human ear, it has been shown that cardiologists are better at detecting significant murmurs than primary-care physicians, and the accuracy of lesion detection by human auscultation is dependent on the valvular lesion.5 To date, AI has not been tested against a cardiologist.
AI-guided ECG interpretation
AI has also emerged as a useful tool in the interpretation of ECGs. Through machine learning, AI is able to recognise patterns in ECGs that are specific to certain types of valvular heart disease, which are currently uninterpretable to the human eye. Kwon et al. developed a deep-learning algorithm based on 39,371 ECGs, coupled with demographic and anthropometric patient details. Of these patients, 1,224 had moderate or severe aortic stenosis (AS) based on echocardiographic data. Once developed, the algorithm was tested on an external validation cohort comprising 10,865 ECGs with the aim of identifying moderate or severe AS. The area under the curve (AUC) was 0.86, with a sensitivity of 80% and specificity of 78.3%. Given the low prevalence of AS in the cohort, the positive-predictive value of the deep-learning algorithm was only 10%, but, importantly, the negative-predictive value was 99%. This would suggest that AI in its current guise would be highly unlikely to miss significant AS based on a patient’s ECG, but would produce a high rate of false positives, which would inevitably lead to further downstream investigation.6 The same group used their deep-learning algorithm to diagnose significant mitral regurgitation (MR) based on ECG, and once again found the model to have high sensitivity (90%) with lower specificity (67%), translating into a negative-predictive value of 99.4% and a positive-predictive value of 10%.7
Ulloa-Cerna et al. have developed a more integrated model using 2,232,130 ECGs coupled with demographic details, in order to diagnose multiple forms of valvular heart disease (including AS, aortic regurgitation [AR], MR, mitral stenosis and tricuspid regurgitation), as well as left ventricular systolic dysfunction (ejection fraction <50%) and interventricular septal hypertrophy (>15 mm). While the predictive value varied according to the pathology, there was a composite 42% positive-predictive value at 90% sensitivity.8 Interestingly, the exact ECG characteristics that are used by AI in order to diagnose valvular heart disease remain incompletely understood; some algorithms appear to place emphasis upon QRS complex morphology, while in others the QT segment, T-wave and T-P segment are more important.9 Nevertheless, the ability of AI to rule out significant valvular heart disease based upon only an ECG could prove to be hugely valuable as a diagnostic and triaging tool.
AI-guided cardiac imaging interpretation
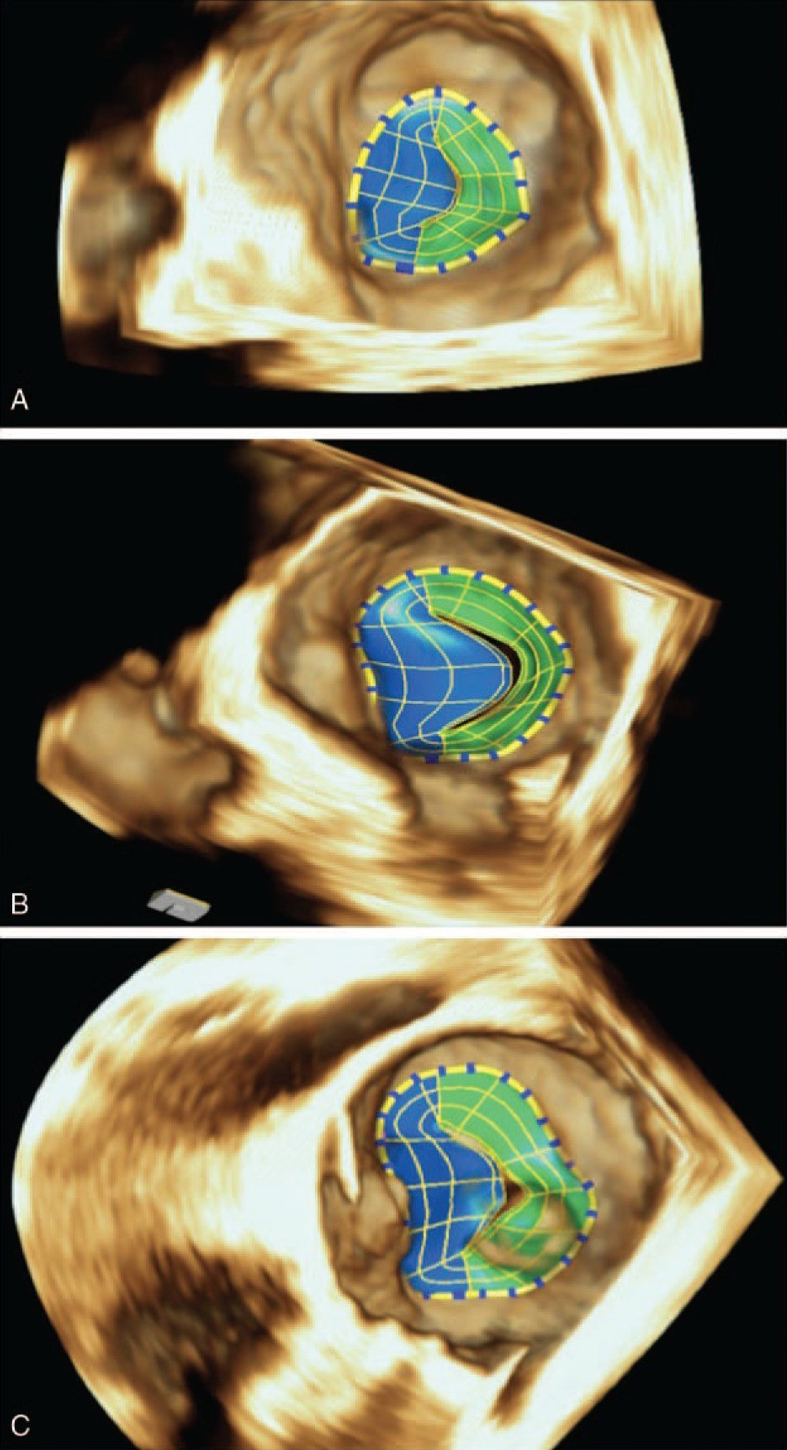
Image taken with permission from Aquila I, Fernández-Golfín C, Rincon LM et al. Fully automated software for mitral annulus evaluation in chronic mitral regurgitation by 3-dimensional transesophageal echocardiography. Medicine 2016;95:e5387. https://doi.org/10.1097/MD.0000000000005387 |
There has been a flurry of automated models for cardiac imaging developed over recent years, with their main utility being in the quantification of disease severity. While manual measurements are typically limited to single points in a cardiac cycle, automated models can process the dynamic movement (i.e. stress) and volume shifts of cardiac structures. This has proven to be particularly useful in determining aetiology and quantifying severity of valvular heart disease. An early example of this was an automated model developed by Grady et al. in 2011, which allowed quantification of MR severity based upon analysis of a 3D echocardiographic image of proximal isovelocity surface area (PISA).10 Subsequently, Aquila et al. (2016) used eSie Valves (Siemens Healthcare, Mountain View, CA, USA) automated software to analyse mitral valve imaging from 3D transoesophageal echocardiography (TOE) in patients with at least moderate MR.11 AI was able to distinguish between primary and secondary MR, and was also able to identify and measure novel parameters that could be used to quantify MR severity, such as change in annular height and distance between mitral annulus and inter-trigonal zone. Such developments have enabled a better understanding of the pathophysiology of mitral valve disease. We now better appreciate differences between primary and secondary MR; in primary MR the mitral annulus enlarges despite preserved mitral annulus function, whereas in secondary MR mitral annulus contractility decreases as it loses its saddle shape and becomes dilated and flattened (figure 1).
AI interpretation of echocardiographic images has also been shown to improve diagnostic accuracy in valvular heart disease when compared with traditional analysis. In patients with at least moderate AR, AI studying 3D full-volume colour Doppler echocardiography (CDE) was compared with standard 2D-PISA CDE with respect to quantifying severity of AR, using phase-contrast cardiac magnetic resonance imaging (PC-CMR) as a benchmark.12 AI more accurately classified AR severity than 2D imaging (k=0.94 vs. k=0.53), particularly in patients with eccentric or multiple regurgitant jets where 90% were graded correctly by AI compared with only 30% by conventional 2D imaging.
AI-guided prognostic predictions
A number of machine-learned prediction models designed to accurately assess the risk of post-procedural mortality have been developed in the past decade (table 1), and in some cases these have been shown to be better able to predict mortality following valvular heart disease intervention than traditional scoring systems, such as EuroSCORE II (which superseded EuroSCORE I in 2012 accommodating for advances in cardiac surgery practice) and Society of Thoracic Surgeons (STS) score. One such model, developed by Hernandez-Suarez et al. using data from 10,883 patients, has shown that artificial neural networks are able to correctly predict post-transcatheter aortic valve implantation (TAVI) in-hospital mortality (i.e. its sensitivity) in 94.4% of cases with an AUC of 0.92.13
Table 1. Artificial intelligence prediction models for mortality after different cardiac interventions
Authors | Patient cohort | Artificial intelligence model (vs. comparator) | Area under curve (AUC) statistic |
Nilsson et al.14 | 4,907 prospective patients undergoing valve-only cardiac surgery | 72 risk factors vs. EuroSCORE model | 0.76 vs. 0.72 for operative mortality |
Celi et al.15 | 165 retrospective patients >80 years old undergoing cardiac surgery | 41 pre-, post- and operative variables vs. EuroSCORE model | 0.941 vs. 0.648 for in-hospital mortality |
Allyn et al.16 | 6,250 retrospective patients undergoing cardiac surgery | 50 variables vs. EuroSCORE II model | 0.795 vs. 0.737 for in-hospital mortality |
Hernandez-Suarez et al.13 | 10,883 retrospective TAVI patients | 43 variables | 0.92 for in-hospital mortality |
Key: TAVI = transcatheter aortic valve implantation |
As hospitals increasingly move towards electronic patient databases, AI provides the potential to develop individualised risk-prediction models based on specific patient characteristics, allowing clinicians to choose the optimal treatment strategy in a more nuanced way than is currently possible. Ultimately, artificial neural networks may be able to compare the risk profile of different treatment strategies, such as surgical aortic valve replacement (SAVR) and TAVI for the treatment of AS, using data specific to a hospital institution, allowing clinicians and patients to make more informed choices.
AI-guided treatment
While a number of the AI models described so far in this paper have the potential to aid diagnosis and treatment of valvular heart disease in the future, some forms of AI are already being used relatively routinely. An example of this is the use of computer-simulation models to aid in the selection of type and size of transcatheter heart valve (THV) prosthesis for TAVI. Traditionally, THV selection involves measurements of the aortic annulus and root using cardiac-gated computed tomography (CT). The main limitation of this approach is that it cannot reliably predict the interaction between the THV and the native anatomy during and following TAVI deployment; in contrast, computer simulations can model the function of different THV types and sizes specific to patient anatomy. In so doing, complications including annular rupture, conduction disturbance, and paravalvular leak can be predicted and, thus, in theory, better avoided. To achieve this, the computer simulation models require deep-learning algorithms that consider the mechanical and geometric properties of the aortic anatomy, as well as the stress-strain relationship associated with varying THVs. Schultz et al. (2016) have shown that using pre-procedural patient simulations of TAVI carried out with various THVs, frame morphology and leaflet calcium displacement can be accurately predicted, when referenced against post-procedural multi-slice CT.17
Now available commercially to clinicians, the FEops HEARTguideTM (FEops NV, Gent, Belgium) can prove invaluable in correctly sizing a THV where there are concerns that cannot be easily resolved using traditional analysis of cardiac-gated CT images. An example of the utility of FEops HEARTguideTM from our institution is a 70-year-old woman with severe, symptomatic AS who was turned down for SAVR due to comorbidities. Analysis of the pre-TAVI CT revealed a left ventricular outflow tract (LVOT) aneurysm, which appeared to involve the annular plane, obscuring the border of the annulus and, thus, making accurate dimension measurements impossible. Due to the difficulty with accurate sizing, and the concern regarding the integrity of the landing zone, a self-expanding THV was selected. The remaining issue was correct size selection, since an oversized valve might have risked annular or aneurysm rupture, while too small a prosthesis risked significant paravalvular leak (PVL). As shown in figure 2, FEops HEARTguideTM was able to predict the contact pressure of various valve sizes and the degree of paravalvular leak at different implantation depths. Based upon our best analysis of the CT images, a 34 mm Evolut Pro+TM (Medtronic, Minneapolis, MN, USA) was our planned THV. However, based upon the FEops HEARTguideTM simulation results, which suggested that use of a 29 mm Medtronic Evolut Pro+TM would result in no excess PVL and lower risk of conduction disturbance, we implanted the smaller valve. This produced an excellent result, with trivial PVL and no conduction disturbance. This case represents a good example of how AI can aid in TAVI by finessing the selection of THV, and guiding implanters with respect to the optimal deployment depth.
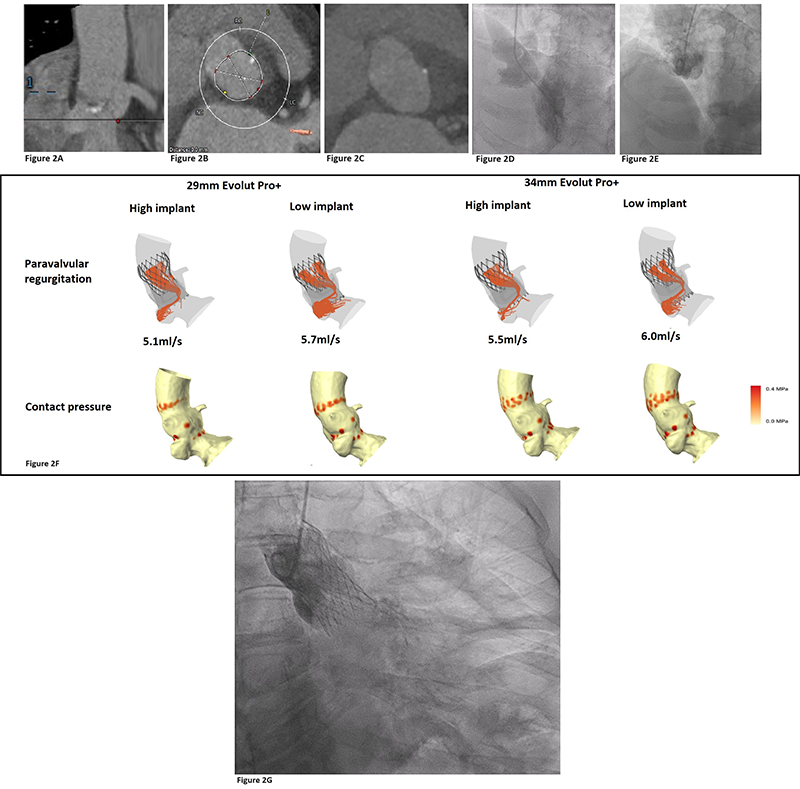
Key: CT = computed tomography; LVOT = left ventricular outflow tract; TAVI = transcatheter aortic valve implant |
Our experience is reflective of existing literature. In a cohort of 33 patients, Hokken and van Mieghem found that AI-based patient simulations resulted in a change to pre-procedural planning in one-third of cases (33%). This yielded better than predicted PVL and reduced pacemaker implantation.18
Another area where AI could play a pivotal role in TAVI relates to ‘lifetime management’. As the indication for TAVI extends into younger patient cohorts,19 a greater number of patients are expected to outlive their THV, necessitating re-treatment in the form of TAVI-in-TAVI. The main concern when considering TAVI-in-TAVI is the threat to coronary perfusion due to the formation of a neo-skirt by displacement of the index THV’s leaflets. If this neo-skirt extends above the sino-tubular junction (STJ), and if the THV frame is in contact with the STJ, the coronaries can essentially be excluded from the circulation – so-called sinus-sequestration. Using traditional means, we can only model this accurately using a CT scan performed after a THV has been implanted. FEops HEARTguideTM modelling can allow physicians to model TAVI-in-TAVI, including the risk of sinus-sequestration, prior to any aortic valve intervention, and, hence, potentially provide guidance as to the most advantageous positioning of the index THV to allow for safe re-treatment.
Utilising AI to guide treatment of other valvular heart disease is still in development. Datasets are currently in development to model the effect of mitral transcatheter edge-to-edge repair on mitral valve regurgitation. As multiple clips are often required during these procedures, obtaining real-time predictions to model the effect of additional clips during a procedure has proven challenging and has limited its clinical utility thus far.20
AI-guided innovation of medical therapies
Taking the concept of AI-guided treatment further, ‘in silico clinical trials’ (ISCT), have the potential to revolutionise the way in which novel therapies are tested and selected for clinical use.21 Currently, the evaluation of any new product requires pre-clinical and clinical trials involving ex vivo tests as well as in vivo tests on animals and humans. The aims of such trials are to demonstrate that a treatment is safe and effective, but this process is costly and time-consuming, while ethical concerns have also been raised. ISCT potentially allows this process to be significantly streamlined, or even replaced entirely, since novel therapies can be tested using computational modelling, rather than in the traditional fashion. Transcatheter valvular heart intervention is an area that is potentially very well suited to ISCT, because almost all patients have pre-procedural CT scans. These, combined with intra-procedural data and post-procedural outcomes, can be used to create a large library of retrospective patient anatomies and physiologies. AI can use this library to generate an almost infinite number of patient anatomies, and new device iterations can be modelled to test safety and effectiveness digitally. As well as being more efficient than bench-top or animal testing, this approach also allows for assessment of long-term device function, including potential modality of failure.
The concept of ISCTs as a means of streamlining the process of bringing novel therapies into clinical practice holds a huge amount of promise, and will be of significant interest to medical device manufacturers and healthcare practitioners alike. The main challenge will likely be regulatory in nature, as the models being used for testing will need to have strict quality control with open data sharing.22
AI-guided robotic surgery
The concept of autonomous robots performing surgical or transcatheter interventions is perhaps the ultimate exemplar of AI involvement in the treatment of valvular heart disease, but is an area that has, so far, been mainly limited to in vivo animal experiments.
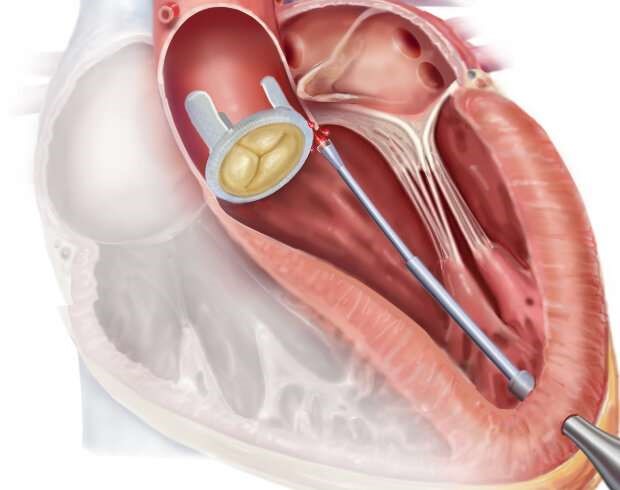
Image courtesy of Fagogenis G, Mencattelli M, Machaidze Z et al. Autonomous robotic intracardiac catheter navigation using haptic vision. Sci Robot 2019;4:aaw1977. https://doi.org/10.1126/scirobotics.aaw1977 |
Currently, use of fully autonomous surgery in clinical practice is not feasible, but the field is developing at a rapid pace. In 2008, using recurrent neural networks with adaptive responses, the Endoscopic Partial-Autonomous Robot (EndoPAR) was shown to be able to perform knot-tying tasks.23 More recently, an algorithm called Motion2Vec learned how to perform suturing from video observations of operators performing the same task.24
The endovascular field has seen even greater automation with the invention of an automated robotic catheter that is capable of identifying PVLs to aid in their closure.25 While traditional PVL closure procedures are performed by clinicians being guided by fluoroscopic and echocardiographic images, the robotic catheter tested by Fagogenis et al. (2019) is able to sense using ‘haptic vision’, combining image and contact receptors (figure 3). In a beating-heart, porcine model, the automated catheter was able to locate an aortic PVL location from the left ventricular apex in 79/83 trials (95% success), and the leak was subsequently closed by a human operator.
The future of AI-guided procedures
Increasing automated involvement in procedures for the treatment of valvular heart disease carries the promise of fewer complications, reduced operating times, and quicker recovery. In order to carry out procedures, robots need to perceive their environment, process this information, and act accordingly. The degree to which a robot can act autonomously has been classified into levels.26 At level 0, the robot is only able to perform actions set by the operator. At level 1, the robot provides different procedural options and can provide manual assistance, such as holding forceps. At present, automation in the medical field is able to operate at level 3, where the robot has conditional autonomy, akin to a self-driving car that can choose and drive the route to a selected destination, and respond to issues arising along the route. By level 4, the robot has gained autonomy but is still under the supervision of the operator, while at level 5, the robot has full autonomy and is not under supervision.
Conclusion
With the advent of AI, we are at the beginning of a new revolution in healthcare that is set to transform the diagnosis and treatment of cardiovascular disease. Our ability to identify and risk stratify patients with valvular heart disease should translate into quicker treatment, with ISCTs resulting in shorter, safer and more effective clinical trials. As AI develops there will likely be safety improvements in valvular heart disease procedures that optimise treatment strategy, specifically tailored to the individual.
Key messages
- Artificial intelligence has been shown to have greater accuracy in diagnosing valvular heart disease, through digital interpretation of heart sounds, electrocardiography (ECG) and echocardiography, than physicians
- Procedural planning of transcatheter valvular therapies has benefited from artificial intelligence with less paravalvular leak and reduced pacemaker implantation seen in transcatheter aortic valve implantation (TAVI)
- Treatment using automation has been shown to be safe and effective in suturing
- In silico clinical trials are set to revolutionise innovation and development of future therapies, likely leading to significant cost and time savings with increased safety and efficacy
Conflicts of interest
PB and AA: none declared. CJM is a proctor for Edwards Lifesciences (Irvine, CA), Medtronic plc. (Minneapolis, MN) and Abbott Vascular (Chicago, IL), as well as receiving honorary speaking fees from Boston Scientific Corporation (Marlborough, MA). MSC has received educational speaking fees from Medtronic plc., Abbott Vascular and Boston Scientific Corporation. DJB is a consultant and proctor for Medtronic plc. and Abbott Vascular, as well as a consultant for Edwards Lifesciences and Boston Scientific. NA has received honorary speaking fees from Medtronic plc. and Abbott Vascular.
Funding
None.
Patient consent
The patient gave full permission for the publication, reproduction, broadcast and other use of photographs, recordings and other audio-visual figures presented in this report.
References
1. Cordeschi R. AI turns fifty: revisiting its origins. Appl Artif Intell 2007;21:259–79. https://doi.org/10.1080/08839510701252304
2. Szolovits P, Pauker SG. Categorical and probabilistic reasoning in medical diagnosis. Artif Intell 1978;11:115–44. https://doi.org/10.1016/0004-3702(78)90014-0
3. LeCun Y, Bengio Y, Hinton G. Deep learning. Nature 2015;521:436–44. https://doi.org/10.1038/nature14539
4. Rancier MA, Israel I, Monickam V, Prince J, Verschoore B, Currie C. Abstract 13244: real world evaluation of an artificial intelligence enabled digital stethoscope for detecting undiagnosed valvular heart disease in primary care. Circulation 2023;148(suppl 1):A13244-A. https://doi.org/10.1161/circ.148.suppl_1.13244
5. Davidsen AH, Andersen S, Halvorsen PA, Schirmer H, Reierth E, Melbye H. Diagnostic accuracy of heart auscultation for detecting valve disease: a systematic review. BMJ Open 2023;13:e068121. https://doi.org/10.1136/bmjopen-2022-068121
6. Kwon JM, Lee SY, Jeon KH et al. Deep learning-based algorithm for detecting aortic stenosis using electrocardiography. J Am Heart Assoc 2020;9:e014717. https://doi.org/10.1161/JAHA.119.014717
7. Kwon J-M, Kim K-H, Akkus Z, Jeon K-H, Park J, Oh B-H. Artificial intelligence for detecting mitral regurgitation using electrocardiography. J Electrocardiol 2020;59:151–7. https://doi.org/10.1016/j.jelectrocard.2020.02.008
8. Ulloa-Cerna AE, Jing L, Pfeifer JM et al. rECHOmmend: an ECG-based machine learning approach for identifying patients at increased risk of undiagnosed structural heart disease detectable by echocardiography. Circulation 2022;146:36–47. https://doi.org/10.1161/CIRCULATIONAHA.121.057869
9. Pandey A, Adedinsewo D. The future of AI-enhanced ECG interpretation for valvular heart disease screening. J Am Coll Cardiol 2022;80:627–30. https://doi.org/10.1016/j.jacc.2022.05.034
10. Grady L, Datta S, Kutter O et al. Regurgitation quantification using 3D PISA in volume echocardiography. In: Fichtinger G, Martel A, Peters T (eds). MICCAI 2011. Lecture Notes in Computer Science, vol 6893. Berlin: Springer, 2011; pp. 512–19. https://doi.org/10.1007/978-3-642-23626-6_63
11. Aquila I, Fernández-Golfín C, Rincon LM et al. Fully automated software for mitral annulus evaluation in chronic mitral regurgitation by 3-dimensional transesophageal echocardiography. Medicine 2016;95:e5387. https://doi.org/10.1097/MD.0000000000005387
12. Choi J, Hong GR, Kim M et al. Automatic quantification of aortic regurgitation using 3D full volume color doppler echocardiography: a validation study with cardiac magnetic resonance imaging. Int J Cardiovasc Imaging 2015;31:1379–89. https://doi.org/10.1007/s10554-015-0707-x
13. Hernandez-Suarez DF, Kim Y, Villablanca P et al. Machine learning prediction models for in-hospital mortality after transcatheter aortic valve replacement. JACC Cardiovasc Interv 2019;12:1328–38. https://doi.org/10.1016/j.jcin.2019.06.013
14. Nilsson J, Ohlsson M, Thulin L, Höglund P, Nashef SA, Brandt J. Risk factor identification and mortality prediction in cardiac surgery using artificial neural networks. J Thorac Cardiovasc Surg 2006;132:12–19. https://doi.org/10.1016/j.jtcvs.2005.12.055
15. Celi LA, Galvin S, Davidzon G, Lee J, Scott D, Mark R. A database-driven decision support system: customized mortality prediction. J Pers Med 2012;2:138–48. https://doi.org/10.3390/jpm2040138
16. Allyn J, Allou N, Augustin P et al. A comparison of a machine learning model with EuroSCORE II in predicting mortality after elective cardiac surgery: a decision curve analysis. PloS One 2017;12:e0169772. https://doi.org/10.1371/journal.pone.0169772
17. Schultz JC, Rodriguez-Olivares R, Bosmans J et al. Patient-specific image-based computer simulation for the prediction of valve morphology and calcium displacement after TAVI with the Medtronic CoreValve and the Edwards SAPIEN valve. EuroIntervention 2016;11:1044–52. https://doi.org/10.4244/EIJV11I9A212
18. Hokken T, Van Mieghem N. CRT-700.26 computed tomography-derived predictive simulations of transcatheter aortic valve replacement in challenging anatomies – the PRECISE TAVI trial. JACC Cardiovasc Interv 2022;15(suppl 4):S61. https://doi.org/10.1016/j.jcin.2022.01.234
19. Coisne A, Lancellotti P, Habib G et al. ACC/AHA and ESC/EACTS guidelines for the management of valvular heart diseases. J Am Coll Cardiol 2023;82:721–34. https://doi.org/10.1016/j.jacc.2023.05.061
20. Dabiri Y, Yao J, Mahadevan VS et al. Mitral valve atlas for artificial intelligence predictions of MitraClip intervention outcomes. Front Cardiovasc Med 2021;8:759675. https://doi.org/10.3389/fcvm.2021.759675
21. Viceconti M, Emili L, Afshari P et al. Possible contexts of use for in silico trials methodologies: a consensus-based review. IEEE J Biomed Health Inform 2021;25:3977–82. https://doi.org/10.1109/JBHI.2021.3090469
22. Rodero C, Baptiste TMG, Barrows RK et al. A systematic review of cardiac in-silico clinical trials. Prog Biomed Eng (Bristol) 2023;5:032004. https://doi.org/10.1088/2516-1091/acdc71
23. Mayer H, Gomez F, Wierstra D, Nagy I, Knoll A, Schmidhuber J. A system for robotic heart surgery that learns to tie knots using recurrent neural networks. Adv Robot 2008;22:1521–37. https://doi.org/10.1163/156855308X360604
24. Tanwani AK, Sermanet P, Yan A, Anand R, Phielipp M, Goldberg K. Motion2vec: semi-supervised representation learning from surgical videos. In: 2020 IEEE International Conference on Robotics and Automation. Paris: 2020; pp. 2174–81. https://doi.org/10.1109/ICRA40945.2020.9197324
25. Fagogenis G, Mencattelli M, Machaidze Z et al. Autonomous robotic intracardiac catheter navigation using haptic vision. Sci Robot 2019;4:aaw1977. https://doi.org/10.1126/scirobotics.aaw1977
26. Yang G-Z, Cambias J, Cleary K et al. Medical robotics – regulatory, ethical, and legal considerations for increasing levels of autonomy. Sci Robot 2017;2:eaam8638. https://doi.org/10.1126/scirobotics.aam8638